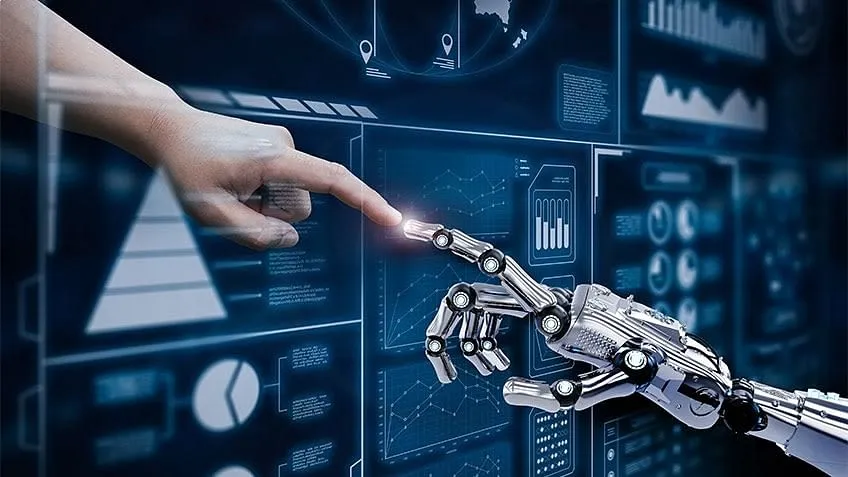
In the realm of technology, one term that has gained unprecedented prominence in recent years is “Machine Learning” (ML). This revolutionary field has not only transformed the way we interact with technology but has also opened new dimensions of possibilities across various industries. In this blog, we’ll embark on a journey to unravel the intricacies of Machine Learning, exploring its origins, applications, and the future it holds.
Understanding the Essence of Machine Learning
The Genesis of Machine Learning
To comprehend the present and future of Machine Learning, it’s essential to trace its roots. Machine Learning, as we know it today, has evolved from the intersection of computer science and statistics. The early concepts of ML date back to the 1940s and 1950s, with pioneers like Alan Turing laying the groundwork for computational learning. However, it wasn’t until the late 20th century that ML gained substantial traction, thanks to advancements in data storage, processing power, and algorithmic development.
Key Components of Machine Learning
Machine Learning operates on a simple yet profound principle: the ability of computers to learn and improve from experience. Three primary components drive this learning process:
- Algorithms: These are the set of instructions that enable machines to perform a specific task or solve a particular problem. In the context of ML, algorithms are designed to learn from data and make predictions or decisions.
- Data: The lifeblood of Machine Learning. Large datasets are fed into ML systems to train them. The quality and quantity of data significantly impact the performance of machine learning models.
- Model: A model is the learned representation of the relationships within the data. It is the outcome of the training process and is used to make predictions on new, unseen data.
Applications of Machine Learning Across Industries
Transforming Healthcare with Predictive Analytics
One of the most impactful applications of Machine Learning is in the healthcare sector. Predictive analytics, a subset of ML, is being employed to forecast disease outbreaks, personalize treatment plans, and improve patient outcomes. Machine Learning algorithms analyze patient data, identify patterns, and provide valuable insights for healthcare professionals, ushering in an era of precision medicine.
Revolutionizing Finance with Fraud Detection
In the financial industry, the ability to detect fraudulent activities is paramount. Machine Learning algorithms excel at sifting through vast amounts of transactional data to identify unusual patterns indicative of fraud. This not only enhances security but also streamlines the customer experience by minimizing false positives and negatives.
Enhancing User Experience in E-Commerce
E-commerce platforms leverage Machine Learning to enhance user experience and drive sales. From personalized product recommendations based on user behavior to dynamic pricing strategies that optimize revenue, ML algorithms continuously adapt and evolve to deliver a seamless and engaging shopping experience.
Autonomous Vehicles and the Future of Transportation
The automotive industry is on the cusp of a transformative era with the integration of Machine Learning into autonomous vehicles. ML algorithms process real-time data from sensors, cameras, and other sources to make split-second decisions, ensuring safe and efficient navigation. The potential impact on road safety and transportation efficiency is staggering.
The Evolving Landscape of Machine Learning
Challenges and Ethical Considerations
As Machine Learning continues to advance, it is not without its challenges. Bias in algorithms, data privacy concerns, and the ethical implications of autonomous decision-making are pressing issues that demand attention. Striking a balance between technological innovation and ethical responsibility is crucial to harness the full potential of Machine Learning.
The Role of Explainability in Machine Learning
Explainability is emerging as a critical aspect of Machine Learning, especially in applications where the decisions made by algorithms have significant consequences. Understanding how and why a machine learning model arrives at a particular decision is essential for building trust and ensuring accountability. Researchers and practitioners are actively working on developing more interpretable ML models to address this need.
Machine Learning in the Quantum Realm
The marriage of Machine Learning and quantum computing holds the promise of solving complex problems that are currently beyond the reach of classical computers. Quantum Machine Learning (QML) explores the synergy between quantum computing’s computational power and ML algorithms, opening up new frontiers in optimization, simulation, and data analysis.
Democratizing Machine Learning with AutoML
As Machine Learning becomes more pervasive, there is a growing need to make it accessible to individuals and organizations with varying levels of technical expertise. AutoML, or Automated Machine Learning, is a step in this direction. It empowers users to automate the end-to-end process of applying ML to real-world problems, democratizing access to the benefits of Machine Learning.
The Future of Machine Learning: A Glimpse into Tomorrow
Advancements in Deep Learning
Deep Learning, a subset of Machine Learning inspired by the structure and function of the human brain, is witnessing rapid advancements. Neural networks with multiple layers (deep neural networks) are unlocking new possibilities in image recognition, natural language processing, and other complex tasks. As computing power continues to grow, the depth and complexity of neural networks will likely follow suit.
Human-AI Collaboration
The future of Machine Learning is not about machines replacing humans but working alongside them. Human-AI collaboration involves harnessing the strengths of both humans and machines to solve problems more efficiently. From healthcare diagnostics to creative endeavors, this collaborative approach has the potential to amplify our capabilities and drive innovation across diverse domains.
Responsible AI and Ethical Frameworks
With the increasing influence of Machine Learning on society, the importance of responsible AI cannot be overstated. Developing and adhering to ethical frameworks is crucial to ensure that ML technologies are deployed in ways that align with human values, avoid discrimination, and promote transparency. The integration of ethics into the design and deployment of ML systems will be a defining factor in shaping the future landscape.
Quantum Machine Learning: A Quantum Leap
Quantum Machine Learning, as a field, is poised to make significant strides in the coming years. The marriage of quantum computing and ML algorithms has the potential to revolutionize industries ranging from finance to drug discovery. As quantum processors become more powerful and accessible, the convergence of quantum and classical computing paradigms will usher in a new era of computational capabilities.
In conclusion, Machine Learning stands at the forefront of technological innovation, reshaping industries and pushing the boundaries of what is possible. From its humble beginnings to the complex algorithms of today, Machine Learning continues to evolve, presenting opportunities and challenges alike. As we look to the future, the responsible and ethical development of Machine Learning will be paramount, ensuring that this transformative technology benefits humanity as a whole. Embracing the marvels of Machine Learning is not just a technological imperative but a collective journey towards a more intelligent and interconnected world.